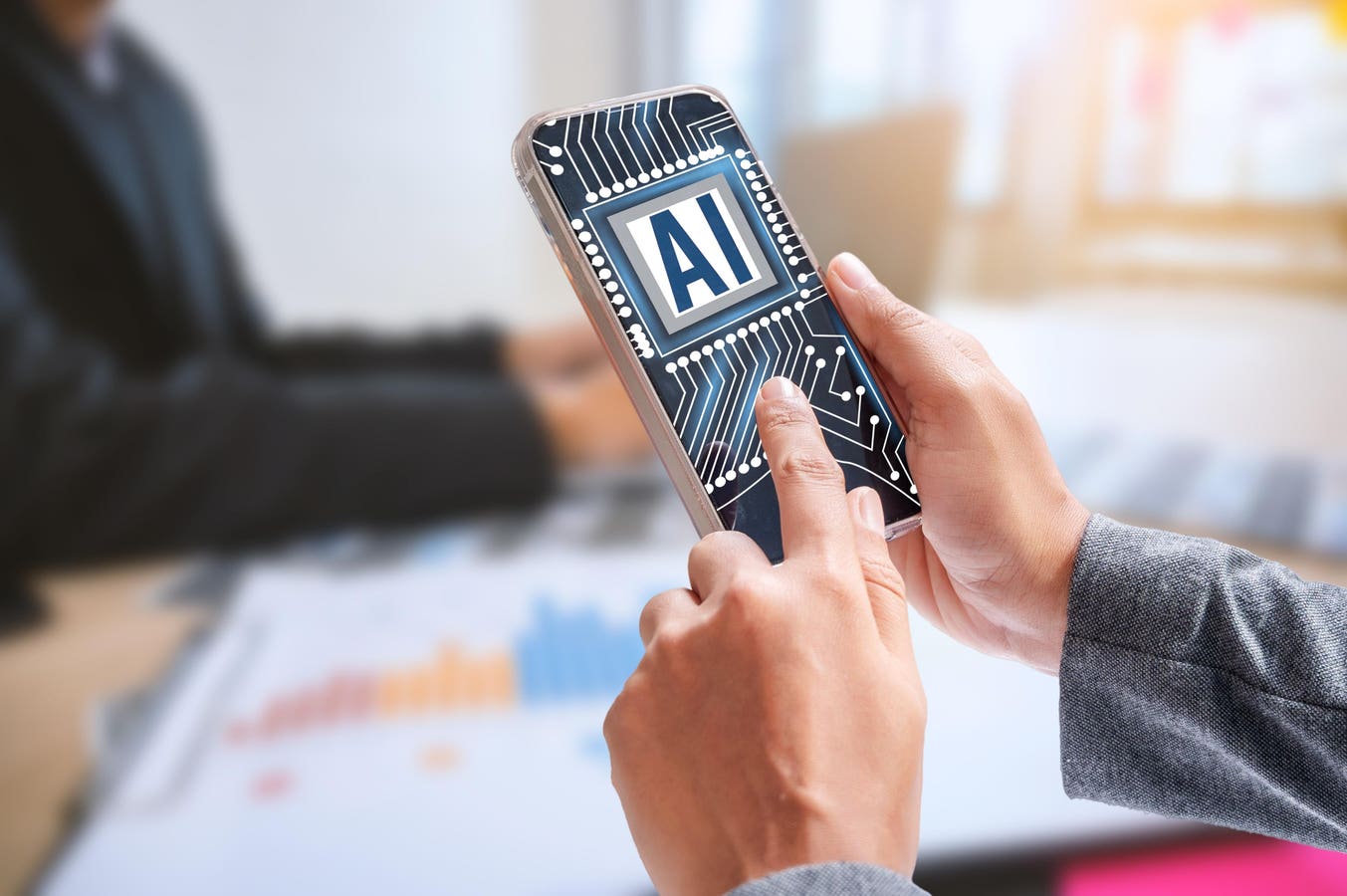
Yadhu Gopalan is the cofounder and CEO of Esper. Esper gives next-gen system administration for company-managed {hardware}.
AI is greater than only a buzzword—it’s a driving pressure behind main technological developments. For companies, early AI adoption is crucial, however correct execution at this time means success tomorrow. Cloud deployments finally introduce points as demand will increase—latency stifles real-time decision-making and knowledge throughput plus computational load drive quickly rising prices. The answer? Run your AI mannequin the place your gadgets are—on the sting.
Why AI At The Edge Is The Future
Conventional AI is cloud-native, the place fashions run, knowledge is processed and outcomes are formulated within the cloud. That is useful for knowledge and resource-heavy AI-processing situations the place latency and price aren’t a difficulty. Edge AI, nevertheless, brings that computation to the place the information is gathered—on-location edge gadgets like smartphones, tablets, kiosks, point-of-sale programs, IoT sensors and comparable. There are a number of compelling benefits of operating AI on the edge versus within the cloud:
• Decrease Latency: As a result of knowledge is created and processed in the identical location, decision-making is finished in actual time, as knowledge doesn’t should be transmitted to and from the cloud. This will dramatically scale back latency, which is crucial for purposes like autonomous automobiles or automated high quality assurance.
• Decreased Prices: This can be a twofold subject: bandwidth and computing prices. As knowledge is transmitted to the cloud (and, in some instances, again), bandwidth utilization will increase. And if you run AI fashions within the cloud, you basically lease sources. Finish service suppliers perceive the worth of computing energy, so this rental comes at an enormous premium. Whenever you run fashions on the sting, you’re utilizing compute energy that you just already personal, so you possibly can considerably scale back bandwidth prices.
• Community Optimizations: Just like price issues for bandwidth, diminished knowledge transmission alleviates the pressure on community infrastructure.
• Enhanced Privateness: Transmitting delicate knowledge all the time poses a minimum of a small threat, so holding that knowledge on a single system or restricted to a neighborhood community reduces the chance of publicity throughout transit.
For the entire advantages of operating AI on the sting, nevertheless, operationalizing can current challenges. Essentially the most important subject comes with AI mannequin deployment. Permit me to clarify.
The AI Mannequin Deployment Problem
Content material supply of all sorts—recordsdata, purposes and system updates, for instance—is a wrestle for a lot of organizations, and AI mannequin deployments solely exacerbate this subject. There are a number of causes for this.
• Configuration Administration: Controlling the setting by which fashions run on the edge is advanced, and also you want tooling designed to make sure the appliance, system and mannequin are configured appropriately. Moreover, having the suitable run time for fashions—and the power to replace the runtime for the {hardware}—is essential.
• {Hardware} Range: When you may have a wide range of gadgets within the subject with totally different computational capabilities and bodily places, AI mannequin deployment at scale is tough to handle.
• Mannequin Replace Frequency: AI fashions are up to date far more often than different varieties of edge content material. If updating month-to-month and even weekly is already a wrestle, day by day or hourly updates are merely out of the query.
• Restricted Sources: Given the {hardware} constraints of most edge gadgets (a minimum of to that of cloud processing), growing dependable AI fashions for native processing with out sacrificing reliability is problematic.
• Dependable Community Infrastructure: Repeatable, scalable software program supply hinges on community reliability, which is difficult for some industries—particularly these working in rural areas.
To beat these challenges, organizations want a complete technique that encompasses the complete AI life cycle, beginning with the gadgets.
The Path Ahead Begins With The {Hardware}
Simply as AI will proceed to affect the best way gadgets are used, your technique round AI mannequin, app and content material distribution additionally has to evolve. Thankfully, an answer already exists on the earth of software program improvement: DevOps.
You could be asking your self what DevOps has to do with system administration. DevOps practices are about alignment between improvement and ops groups, and lengthening that idea past software program improvement to the sting is the place the magic occurs. With a DevOps philosophy utilized to system administration, your improvement and IT groups can work collectively to construct, take a look at, apply and iterate AI fashions (or another sort of content material).
Utilizing trendy instruments and expertise offered by forward-thinking system administration options, this isn’t a theoretical dialog, both. With instruments like distribution pipelines, testing environments and staged software program updates, AI mannequin distribution can turn out to be a non-issue. This frees your improvement crew to work on future updates, your IT crew to maneuver with agility and your corporation to concentrate on what’s vital.
Forbes Technology Council is an invitation-only neighborhood for world-class CIOs, CTOs and expertise executives. Do I qualify?